3A - Methods
Tracks
Track 1
Friday, July 18, 2025 |
10:30 AM - 12:00 PM |
Grand Room 1 |
Speaker
Dr Jessica Cameron
Senior Research Fellow
Cancer Council Queensland
Spatial estimates of cancer-specific mortality and survival provide different interpretations
Abstract
Background
Spatial modelling of cancer survival has revealed substantial disparities in the prognosis of people diagnosed with cancer in Australia. Mortality and survival are two related measures often used to convey disease burden and prognosis. However, they measure very different properties, providing different interpretations about outcomes. Relative survival describes risk of death in a cancer cohort, adjusting for non-cancer deaths. Cancer-specific mortality measures population-level risk of death. Here, we demonstrate the differences and issues through spatial modelling.
Methods
Individual-level cancer diagnosis and cause-specific mortality records were obtained from the Queensland Cancer Register.
Bayesian spatial incidence, mortality and 5-year relative survival models were fitted to the observations for lung cancer (ICD-10: C33-C34), melanoma (C43) and prostate cancer (C61). Area-level rates were compared with the state average for each outcome.
Results
Geographic patterns of survival differed from those of cancer-specific mortality for each cancer type. Together, these measures provided differing insights depending on the type of cancer. For prostate cancer, most areas’ mortality rates were similar to the Queensland average and areas where survival was better than average were in major cities and also had higher than average incidence rates. For melanoma, most areas’ survival rates were similar to the state average and mortality rates tended to be correlated with incidence rates. For lung cancer, all three measures were correlated.
Conclusion
Cancer mortality and survival measures provide complementary insights into geographic disparities in cancer deaths. Disparities in cancer-specific mortality rates may reflect differences in incidence rates or poorer longer-term survival but depend on accurate cause-of-death coding. By contrast, relative survival rates can also reflect lead time, as well as deaths indirectly attributable to the cancer diagnosis, such as sequalae of the cancer and its treatment. Both measures, along with incidence, are required to fully assess the impact of cancer on a population.
Spatial modelling of cancer survival has revealed substantial disparities in the prognosis of people diagnosed with cancer in Australia. Mortality and survival are two related measures often used to convey disease burden and prognosis. However, they measure very different properties, providing different interpretations about outcomes. Relative survival describes risk of death in a cancer cohort, adjusting for non-cancer deaths. Cancer-specific mortality measures population-level risk of death. Here, we demonstrate the differences and issues through spatial modelling.
Methods
Individual-level cancer diagnosis and cause-specific mortality records were obtained from the Queensland Cancer Register.
Bayesian spatial incidence, mortality and 5-year relative survival models were fitted to the observations for lung cancer (ICD-10: C33-C34), melanoma (C43) and prostate cancer (C61). Area-level rates were compared with the state average for each outcome.
Results
Geographic patterns of survival differed from those of cancer-specific mortality for each cancer type. Together, these measures provided differing insights depending on the type of cancer. For prostate cancer, most areas’ mortality rates were similar to the Queensland average and areas where survival was better than average were in major cities and also had higher than average incidence rates. For melanoma, most areas’ survival rates were similar to the state average and mortality rates tended to be correlated with incidence rates. For lung cancer, all three measures were correlated.
Conclusion
Cancer mortality and survival measures provide complementary insights into geographic disparities in cancer deaths. Disparities in cancer-specific mortality rates may reflect differences in incidence rates or poorer longer-term survival but depend on accurate cause-of-death coding. By contrast, relative survival rates can also reflect lead time, as well as deaths indirectly attributable to the cancer diagnosis, such as sequalae of the cancer and its treatment. Both measures, along with incidence, are required to fully assess the impact of cancer on a population.
Dr Benjamin Daniels
Senior Research Fellow
School Of Population Health, Unsw Sydney
Robust real-world evidence for Australian medicines use: A blinded, multi-centre replication experiment
Abstract
Background
Routinely-collected health data require extensive preparation when used to generate real-world evidence (RWE). Decisions made by different analysts conducting the same intended analysis may lead to results that do not replicate. We conducted an experiment examining the impacts of these decisions on the use the diabetes medicine, metformin, in Australian clinical practice.
Methods
Our four sites independently developed a HARmonized Protocol Template to Enhance Reproducibility (HARPER) protocol based on the same master protocol and executed analyses using the same Pharmaceutical Benefits Scheme 10% sample dataset of medicine dispensing records from 2015 to 2019. These data include the strength and quantity of the medicine dispensed, but not the intended dose or duration of treatment. Each site calculated: cohort size and demographics; periods of exposure; and treatment outcome events including discontinuation, switching to another diabetes medicine, intensification (addition of another diabetes treatment); and associations between cohort characteristics and time to each treatment event. We assessed concordance by site by measuring deviations from the calculated median value across the sites.
Results
We observed good agreement across all sites for the number of people initiating treatment (median: 53,127, range: 51,848-55,273), gender (median: 56.9% female, range: 56.8-57.1%) and age group. However, each site employed different methods for estimating periods of exposure and used different operational definitions for treatment events. Consequently, we found poor agreement for the one-year incidence of discontinuation (median: 55%, range: 34-67%), switching (median: 3.5%, range: 1-7%), intensification (median: 8%, range: 5-12%), time to event estimates and hazard ratios.
Conclusions
Different analytical decisions when deriving exposure from dispensing data impact replicability. More specific detail in harmonising approaches to defining exposure periods and standardised concepts of treatment events will enhance the quality of RWE in medicines utilisation research.
Routinely-collected health data require extensive preparation when used to generate real-world evidence (RWE). Decisions made by different analysts conducting the same intended analysis may lead to results that do not replicate. We conducted an experiment examining the impacts of these decisions on the use the diabetes medicine, metformin, in Australian clinical practice.
Methods
Our four sites independently developed a HARmonized Protocol Template to Enhance Reproducibility (HARPER) protocol based on the same master protocol and executed analyses using the same Pharmaceutical Benefits Scheme 10% sample dataset of medicine dispensing records from 2015 to 2019. These data include the strength and quantity of the medicine dispensed, but not the intended dose or duration of treatment. Each site calculated: cohort size and demographics; periods of exposure; and treatment outcome events including discontinuation, switching to another diabetes medicine, intensification (addition of another diabetes treatment); and associations between cohort characteristics and time to each treatment event. We assessed concordance by site by measuring deviations from the calculated median value across the sites.
Results
We observed good agreement across all sites for the number of people initiating treatment (median: 53,127, range: 51,848-55,273), gender (median: 56.9% female, range: 56.8-57.1%) and age group. However, each site employed different methods for estimating periods of exposure and used different operational definitions for treatment events. Consequently, we found poor agreement for the one-year incidence of discontinuation (median: 55%, range: 34-67%), switching (median: 3.5%, range: 1-7%), intensification (median: 8%, range: 5-12%), time to event estimates and hazard ratios.
Conclusions
Different analytical decisions when deriving exposure from dispensing data impact replicability. More specific detail in harmonising approaches to defining exposure periods and standardised concepts of treatment events will enhance the quality of RWE in medicines utilisation research.
Mr Harrison Hansford
Phd Candidate
Unsw Sydney
Transparent Reporting of Observational Studies Emulating a Target Trial: The TARGET Guideline
Abstract
BACKGROUND
When randomised trials are not available or feasible, real-world observational data can be used to answer causal questions about the effects of interventions by emulating a hypothetical, randomised trial (target trial). However, inconsistent and incomplete reporting in published studies using the target trial framework has been identified. We developed consensus-based guidance estimating causal effects by explicitly emulating a target trial.
METHODS
We developed the TARGET (Transparent Reporting of Observational Studies Emulating a Target Trial) guideline following methodological guidance from the EQUATOR Network. This included a systematic review of reporting practices in studies explicitly aiming to emulate a target trial; a 2-round online survey (Aug 2023-Mar 2024; 18 expert participants) to identify and refine items selected from previous research; an expert consensus meeting (Jun 2024; 18 panellists) to refine the scope of the guideline and draft the checklist; and an external piloting activity with stakeholders (n=66; Sept 2024-Jan 2025). We piloted and revised the checklist.
RESULTS
TARGET provides a minimum set of items that should be reported in observational studies of interventions explicitly emulating a target trial. The 20-item TARGET checklist has 6 sections (abstract, introduction, methods, results, discussion & additional information). Key recommendations are (1) the causal question should be stated, including the reason for emulating a target trial, (2) the target trial protocol should be clearly specified and how the protocol was mapped to the observational data should be thoroughly described, and (3) for each causal estimand, the estimate obtained and its precision should be reported along with findings from additional analyses to assess robustness to potential violations of assumptions, design and analysis choices.
DISCUSSION
Use of TARGET should facilitate transparent reporting of studies explicitly emulating a target trial to improve peer review, support pharmaco/epidemiologists, clinicians and policymakers designing and/or interpreting these studies.
When randomised trials are not available or feasible, real-world observational data can be used to answer causal questions about the effects of interventions by emulating a hypothetical, randomised trial (target trial). However, inconsistent and incomplete reporting in published studies using the target trial framework has been identified. We developed consensus-based guidance estimating causal effects by explicitly emulating a target trial.
METHODS
We developed the TARGET (Transparent Reporting of Observational Studies Emulating a Target Trial) guideline following methodological guidance from the EQUATOR Network. This included a systematic review of reporting practices in studies explicitly aiming to emulate a target trial; a 2-round online survey (Aug 2023-Mar 2024; 18 expert participants) to identify and refine items selected from previous research; an expert consensus meeting (Jun 2024; 18 panellists) to refine the scope of the guideline and draft the checklist; and an external piloting activity with stakeholders (n=66; Sept 2024-Jan 2025). We piloted and revised the checklist.
RESULTS
TARGET provides a minimum set of items that should be reported in observational studies of interventions explicitly emulating a target trial. The 20-item TARGET checklist has 6 sections (abstract, introduction, methods, results, discussion & additional information). Key recommendations are (1) the causal question should be stated, including the reason for emulating a target trial, (2) the target trial protocol should be clearly specified and how the protocol was mapped to the observational data should be thoroughly described, and (3) for each causal estimand, the estimate obtained and its precision should be reported along with findings from additional analyses to assess robustness to potential violations of assumptions, design and analysis choices.
DISCUSSION
Use of TARGET should facilitate transparent reporting of studies explicitly emulating a target trial to improve peer review, support pharmaco/epidemiologists, clinicians and policymakers designing and/or interpreting these studies.
Dr Madeleine Hinwood
Statistician/Epidemiologist
Hunter Medical Research Institute & The University of Newcastle
Using multiple estimands to understand treatment effects with competing risks
Abstract
Background: In pharmacoepidemiological studies with long follow-up, competing events (primarily all-cause mortality) can complicate effect estimation. Single effect measures may not capture the full complexity of treatment effects in the presence of strong competing risks. We demonstrate the value of multiple estimands using a case study of medication effects on dementia risk after stroke, though the approach is broadly applicable to many epidemiological settings where competing events are common.
Methods: Using Swedish national registry data (n=125,865), we emulated a target trial examining the effects of common antiplatelet drugs, P2Y12 receptor inhibitors, on dementia risk after stroke. We estimated both total effects (capturing the real-world impact of treatment on dementia via any path, including those mediated by death) and the controlled direct effect (estimating treatment effect in a scenario where death was prevented). We compared these estimands to understand how competing risks influenced treatment effects, using inverse probability weighting to adjust for confounding.
Results: The two estimands revealed different aspects of the treatment effect. The total effect showed a modest reduction in 5-year dementia risk associated with treatment (-1.07 percentage points [95% CI: -1.58, -0.47]). The controlled direct effect, estimating the treatment effect if death had been prevented, demonstrated a larger protective effect (-2.23 percentage points [95% CI: -2.78, -1.69]). This difference highlights how the competing risk of all-cause mortality partially masked the cognitive benefits of treatment.
Conclusion: Multiple estimands provide complementary insights in the presence of competing risks. Total effects inform real-world decision-making, while the controlled direct effect helps understand exposure-outcome pathways and provide an understanding of biological mechanisms. This approach extends beyond aging populations to any setting with competing events, such as perinatal or oncological research, demonstrating how modern causal inference methods can address complex epidemiological questions.
Methods: Using Swedish national registry data (n=125,865), we emulated a target trial examining the effects of common antiplatelet drugs, P2Y12 receptor inhibitors, on dementia risk after stroke. We estimated both total effects (capturing the real-world impact of treatment on dementia via any path, including those mediated by death) and the controlled direct effect (estimating treatment effect in a scenario where death was prevented). We compared these estimands to understand how competing risks influenced treatment effects, using inverse probability weighting to adjust for confounding.
Results: The two estimands revealed different aspects of the treatment effect. The total effect showed a modest reduction in 5-year dementia risk associated with treatment (-1.07 percentage points [95% CI: -1.58, -0.47]). The controlled direct effect, estimating the treatment effect if death had been prevented, demonstrated a larger protective effect (-2.23 percentage points [95% CI: -2.78, -1.69]). This difference highlights how the competing risk of all-cause mortality partially masked the cognitive benefits of treatment.
Conclusion: Multiple estimands provide complementary insights in the presence of competing risks. Total effects inform real-world decision-making, while the controlled direct effect helps understand exposure-outcome pathways and provide an understanding of biological mechanisms. This approach extends beyond aging populations to any setting with competing events, such as perinatal or oncological research, demonstrating how modern causal inference methods can address complex epidemiological questions.
Ms Samantha Howe
PhD Student & Research Assistant
University Of Melbourne
Modelling the health and economic impacts of a tobacco-free-generation policy in Australia
Abstract
Introduction
Some states in Australia are currently considering implementing a ‘tobacco free generation (TFG)’ policy, to phase out tobacco supply to future generations. This project aims to quantify the future health and economic (healthcare perspective) impacts of a TFG policy implemented at the national level, in comparison to both business-as-usual (BAU) and a less radical ‘T21’ policy in which the legal age of purchasing tobacco is increased to 21.
Methods
A Markov process was constructed to simulate future smoking behaviours in the Australian population, with a proportional multistate lifetable (PMSLT) that sums the health impacts of 31 smoking-related diseases. The model outputs the difference in deaths, health-adjusted life years (HALYs), and disease expenditure, for the Australian population under the two interventions compared to ‘business-as-usual’ (BAU), over 20-60 years. The TFG was parameterised to reduce smoking uptake by 90% compared to BAU over 10-years, and the T21 policy parameterised using existing policy analyses from the US.
Results
In comparison to BAU, the TFG policy is expected to result in an estimated 56,000 HALYs being gained, and 5,200 deaths being averted, between 2025-2065, yielding approximately four times the health gains under the T21 policy. Across sociodemographic groups, the largest health gains under the TFG were for the remote and most disadvantaged populations. The TFG policy additionally resulted in $180 million in healthcare savings over 20-years, compared to $52 million under the T21 policy.
Conclusions
Our findings show the potential population health gains under TFG policy in Australia and highlight the importance of having equity at the forefront of tobacco control policy. While a TFG policy should be considered in Australia, though it is important that these occur in conjunction with efforts to reduce existing smoking rates (and produce more immediate population health gains) through novel efforts targeting smoking cessation.
Some states in Australia are currently considering implementing a ‘tobacco free generation (TFG)’ policy, to phase out tobacco supply to future generations. This project aims to quantify the future health and economic (healthcare perspective) impacts of a TFG policy implemented at the national level, in comparison to both business-as-usual (BAU) and a less radical ‘T21’ policy in which the legal age of purchasing tobacco is increased to 21.
Methods
A Markov process was constructed to simulate future smoking behaviours in the Australian population, with a proportional multistate lifetable (PMSLT) that sums the health impacts of 31 smoking-related diseases. The model outputs the difference in deaths, health-adjusted life years (HALYs), and disease expenditure, for the Australian population under the two interventions compared to ‘business-as-usual’ (BAU), over 20-60 years. The TFG was parameterised to reduce smoking uptake by 90% compared to BAU over 10-years, and the T21 policy parameterised using existing policy analyses from the US.
Results
In comparison to BAU, the TFG policy is expected to result in an estimated 56,000 HALYs being gained, and 5,200 deaths being averted, between 2025-2065, yielding approximately four times the health gains under the T21 policy. Across sociodemographic groups, the largest health gains under the TFG were for the remote and most disadvantaged populations. The TFG policy additionally resulted in $180 million in healthcare savings over 20-years, compared to $52 million under the T21 policy.
Conclusions
Our findings show the potential population health gains under TFG policy in Australia and highlight the importance of having equity at the forefront of tobacco control policy. While a TFG policy should be considered in Australia, though it is important that these occur in conjunction with efforts to reduce existing smoking rates (and produce more immediate population health gains) through novel efforts targeting smoking cessation.
Dr Kerry Staples
Senior Analyst And Research Officer
Department Of Health, Western Australia
Becoming Bayesian: From innovative technique to business as usual, building staff capacity
Abstract
Background
Western Australian local government authorities (LGAs) are required by law to produce public health plans. To be locally specific, each needs data for hospitalisation and mortality, infectious disease notifications, and health condition and risk factor prevalence. Thirty percent of LGAs have fewer than 1,000 residents, limiting data provision due to small case numbers. Bayesian methods are becoming more widely used for similar epidemiological data and could help us fill data gaps. It was necessary to upskill and build staff capacity in this complex area so we could incorporate this method into our core business.
Methods
We partnered with university researchers and took a structured approach to building staff capacity for both theoretical and practical Bayesian analysis utilising:
• A facilitated 3-day training workshop.
• Fortnightly meeting to discuss progress and technical issues.
• Learning materials, written training materials, sample scripts and recorded training sessions.
• Ad-hoc technical support provided as issues arose.
Results
Two Bayesian spatio-temporal models were developed that allowed LGA profile production for all 137 LGAs without data gaps. Additionally, epidemiological indicators from population survey, cancer incidence, potentially preventable hospitalisations and burden of disease were modelled at the LGA level. Heat-related and environmentally-related hospitalisations are scheduled to be completed soon.
Staff capacity has been developed allowing us to tackle many theoretical and practical challenges, such as changing processes to accommodate different data distributions. Other skills developed are utilising, explaining, and budgeting for complex computing needs such as local and remote high-performance computing resources. These skills are valuable and not often developed in formal epidemiological training.
Conclusion
Capacity building is essential for effective integration of innovative epidemiological methods to core business. Staff training, learning materials and supported problem-solving has allowed us to embed Bayesian analysis as core business, allowing more flexible data provision to support public health planning.
Western Australian local government authorities (LGAs) are required by law to produce public health plans. To be locally specific, each needs data for hospitalisation and mortality, infectious disease notifications, and health condition and risk factor prevalence. Thirty percent of LGAs have fewer than 1,000 residents, limiting data provision due to small case numbers. Bayesian methods are becoming more widely used for similar epidemiological data and could help us fill data gaps. It was necessary to upskill and build staff capacity in this complex area so we could incorporate this method into our core business.
Methods
We partnered with university researchers and took a structured approach to building staff capacity for both theoretical and practical Bayesian analysis utilising:
• A facilitated 3-day training workshop.
• Fortnightly meeting to discuss progress and technical issues.
• Learning materials, written training materials, sample scripts and recorded training sessions.
• Ad-hoc technical support provided as issues arose.
Results
Two Bayesian spatio-temporal models were developed that allowed LGA profile production for all 137 LGAs without data gaps. Additionally, epidemiological indicators from population survey, cancer incidence, potentially preventable hospitalisations and burden of disease were modelled at the LGA level. Heat-related and environmentally-related hospitalisations are scheduled to be completed soon.
Staff capacity has been developed allowing us to tackle many theoretical and practical challenges, such as changing processes to accommodate different data distributions. Other skills developed are utilising, explaining, and budgeting for complex computing needs such as local and remote high-performance computing resources. These skills are valuable and not often developed in formal epidemiological training.
Conclusion
Capacity building is essential for effective integration of innovative epidemiological methods to core business. Staff training, learning materials and supported problem-solving has allowed us to embed Bayesian analysis as core business, allowing more flexible data provision to support public health planning.
Dr Heidi Welberry
Lecturer
University Of New South Wales
Novel methods to investigate trends in Australian dementia risk profiles over time
Abstract
Background: Modifiable dementia risk factors often coexist in individuals. Understanding the combined risk of multiple factors is crucial for targeted prevention. Traditional methods for calculating a combined population attributable fraction (PAF) rely on assumptions such as independence of risk factors. We recently enhanced these methods by calculating attributable risk at the individual level and directly incorporating this into population-level estimates. This accounts for complex individual-level risk factor clustering while supporting flexible assumptions about the way risk accumulates. We apply this approach to modifiable dementia risk factors in Australia to investigate trends over time.
Methods: We calculated prevalence for 12 risk factors (low education, hypertension, obesity, high cholesterol, smoking, high alcohol, poor diet, physical inactivity, hearing loss, depression, diabetes, and social isolation), using five national Australian health surveys: 2007/8 to 2022. Adjusted prevalence ratios and combined PAFs were estimated. Population sub-groups were defined by sex and socio-economic disadvantage (lowest 40% household income versus highest 60%). Results were disaggregated by life-stage (mid-life: 45-64 years; late-life: 65-84 years).
Results: Mid-life smoking, high alcohol, physical inactivity, hearing loss and low education decreased; obesity, depression and poor diet increased; resulting in no change in combined PAF: 47.2% (46.5-48.0) in 2007/8 and 46.9% in 2022 (45.9-47.7). Late-life high alcohol, physical inactivity and low education decreased; depression and poor diet increased; with no change in combined PAF: 51.5% (50.9-52.5) in 2007/8 and 51.4% (50.7-52.4) in 2022. In mid-life, modifiable risk was higher among low-income groups and males and depression was the leading modifiable risk factor in 2022, disproportionately affecting low-income households and females.
Conclusions: The modifiable PAF for dementia in Australia in the last 15 years remained stable, but the profile of risk has changed. Low-income groups (compared to high) have substantially higher modifiable risk and tailored support targeted towards areas of disadvantage may help reduce disparities.
Methods: We calculated prevalence for 12 risk factors (low education, hypertension, obesity, high cholesterol, smoking, high alcohol, poor diet, physical inactivity, hearing loss, depression, diabetes, and social isolation), using five national Australian health surveys: 2007/8 to 2022. Adjusted prevalence ratios and combined PAFs were estimated. Population sub-groups were defined by sex and socio-economic disadvantage (lowest 40% household income versus highest 60%). Results were disaggregated by life-stage (mid-life: 45-64 years; late-life: 65-84 years).
Results: Mid-life smoking, high alcohol, physical inactivity, hearing loss and low education decreased; obesity, depression and poor diet increased; resulting in no change in combined PAF: 47.2% (46.5-48.0) in 2007/8 and 46.9% in 2022 (45.9-47.7). Late-life high alcohol, physical inactivity and low education decreased; depression and poor diet increased; with no change in combined PAF: 51.5% (50.9-52.5) in 2007/8 and 51.4% (50.7-52.4) in 2022. In mid-life, modifiable risk was higher among low-income groups and males and depression was the leading modifiable risk factor in 2022, disproportionately affecting low-income households and females.
Conclusions: The modifiable PAF for dementia in Australia in the last 15 years remained stable, but the profile of risk has changed. Low-income groups (compared to high) have substantially higher modifiable risk and tailored support targeted towards areas of disadvantage may help reduce disparities.
Dr Rushani Wijesuriya
Research Officer (Biostatistician)
Murdoch Children's Research Institute
Simultaneous assessment of multiple biases in causal inference
Abstract
Background: Epidemiological studies aiming to quantify the causal effect of an exposure on an outcome generally rely on unverifiable assumptions, which if violated can induce systematic bias such as selection, measurement, and confounding bias. Quantitative bias analysis methods allow assessing robustness of results to assumption violations, by producing bias-adjusted estimates under alternative assumptions. However, flexible and accessible methods for simultaneous multiple bias analysis are lacking. Except for a recent approach that relies on inverse probability of selection weighting, existing methods for individual participant data bias analysis can only adjust for a single source of bias at a time, ignoring others, which does not reflect the overall impact of potential biases on the causal effect estimate.
Methods: Although characterised as distinct types, fundamentally each bias can be viewed as emanating from a missing data problem. In this project we use this framework to develop a novel bias analysis method using missing data imputation. Specifically, we use fully conditional specification-based multiple imputation approach to obtain estimates that are simultaneously adjusted for multiple biases. We conducted a simulation study to evaluate the method’s performance and compare it to the existing approach that uses inverse probability of selection weighting and illustrate its practical value in the analysis of a case study investigating the effect of breastfeeding on risk of childhood asthma.
Results: Preliminary simulations show that the multiple imputation-based approach results in approximately unbiased causal effect estimates under alternative assumptions
Conclusions: Multiple imputation-based approaches can be used in practice to flexibly assess the overall impact of multiple potential biases.
Methods: Although characterised as distinct types, fundamentally each bias can be viewed as emanating from a missing data problem. In this project we use this framework to develop a novel bias analysis method using missing data imputation. Specifically, we use fully conditional specification-based multiple imputation approach to obtain estimates that are simultaneously adjusted for multiple biases. We conducted a simulation study to evaluate the method’s performance and compare it to the existing approach that uses inverse probability of selection weighting and illustrate its practical value in the analysis of a case study investigating the effect of breastfeeding on risk of childhood asthma.
Results: Preliminary simulations show that the multiple imputation-based approach results in approximately unbiased causal effect estimates under alternative assumptions
Conclusions: Multiple imputation-based approaches can be used in practice to flexibly assess the overall impact of multiple potential biases.
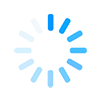