1A -Big Data
Tracks
Track 1
Thursday, July 17, 2025 |
10:30 AM - 12:00 PM |
Grand Room 1 |
Speaker
Miss Xuemei Zhang
Research Assistant
University of Melbourne
A Lifelong Journey to Combat Non-Communicable Diseases
Abstract
Background
Non-communicable diseases (NCDs) are the leading causes of morbidity and mortality globally. NCDs commonly happen in adulthood, while evidence suggests their roots may be traced back to early life stages. However, existing studies mostly examined childhood or adulthood socioeconomic status (SES) independently. This study aims to explore the complex interactions between NCDs development, childhood SES, adulthood SES, and SEE mobility among adults in China.
Method
This study used longitudinal data obtained from nationally representative surveys-China Family Panel Studies (CFPS). Participants aged 25 years and older were included, with self-reported doctor-diagnosed NCDs used as the outcome variable. Participants reported their own and their parents’ highest level of education was used as the proxy of adulthood and childhood SES. Childhood SES and adulthood SES were categorized into two groups (low vs high) based on the median of the highest level of education of respondents and the SES mobility variable was then constructed comprised of four categories: stable low, upward, downward, and stable high. Multivariable logistic regression models were fitted for investigating the relationship between NCD and different SES and all analysis is stratified by gender and age.
Results
We finally included 25,167 participants. 67.60% of the participants are in the ‘stable low’ category. Participants who have a higher childhood SES (14.25%), and higher adulthood SES (13.92%) have higher NCD prevalence. Participants experienced upward mobility (15.03%) had the highest NCD prevalence. There is a significant positive relationship between childhood SES and adulthood SES and NCD prevalence is more significant in the older cohorts and reversed in the younger cohorts.
Conclusion
Adulthood SES has a more significant effect on the development of NCD, while childhood situation has a certain lag in its impact on NCDs. Mobility does not play a significant role in the development of disease, except in the older age groups.
Non-communicable diseases (NCDs) are the leading causes of morbidity and mortality globally. NCDs commonly happen in adulthood, while evidence suggests their roots may be traced back to early life stages. However, existing studies mostly examined childhood or adulthood socioeconomic status (SES) independently. This study aims to explore the complex interactions between NCDs development, childhood SES, adulthood SES, and SEE mobility among adults in China.
Method
This study used longitudinal data obtained from nationally representative surveys-China Family Panel Studies (CFPS). Participants aged 25 years and older were included, with self-reported doctor-diagnosed NCDs used as the outcome variable. Participants reported their own and their parents’ highest level of education was used as the proxy of adulthood and childhood SES. Childhood SES and adulthood SES were categorized into two groups (low vs high) based on the median of the highest level of education of respondents and the SES mobility variable was then constructed comprised of four categories: stable low, upward, downward, and stable high. Multivariable logistic regression models were fitted for investigating the relationship between NCD and different SES and all analysis is stratified by gender and age.
Results
We finally included 25,167 participants. 67.60% of the participants are in the ‘stable low’ category. Participants who have a higher childhood SES (14.25%), and higher adulthood SES (13.92%) have higher NCD prevalence. Participants experienced upward mobility (15.03%) had the highest NCD prevalence. There is a significant positive relationship between childhood SES and adulthood SES and NCD prevalence is more significant in the older cohorts and reversed in the younger cohorts.
Conclusion
Adulthood SES has a more significant effect on the development of NCD, while childhood situation has a certain lag in its impact on NCDs. Mobility does not play a significant role in the development of disease, except in the older age groups.
Ms Aimée Altermatt
Research Assistant
Burnet Institute
Propensity score estimation: assessing machine learning methods for an observational prison cohort
Abstract
Background
People recently released from prison experience poor health outcomes and evidence-based public health policy is critical to improve their health. Causal evidence for these policies can be found when exposure groups are balanced on all measured covariates, as in a randomised controlled trial. In observational studies, propensity scores are estimated to reweight data to achieve covariate balance. Using data from an observational study of incarcerated men, we compared how machine learning and traditional logistic regression propensity estimation methods perform in obtaining balanced datasets.
Methods
We evaluated three propensity estimation methods: logistic regression, gradient boosting and random forest. The resulting covariate balance was assessed using the average standardised absolute mean difference (ASAM; a lower ASAM indicates better balance between groups). For each method, we fit a causal model with inverse probability weighting to estimate causal risk difference and 95% prediction interval (95%PI). Data were from an observational cohort study of imprisoned men with survey and administrative data pre- and post-release. The exposure was self-reported access to emotional support whilst in prison (yes/no). The outcome was emergency department (ED) presentation within 100 days post prison release (yes/no), derived from administrative data.
Results
Of 326 men, 229 (70%) reported accessing emotional support whilst imprisoned; 75 (23%) attended ED within 100 days post release. ASAM in the unweighted sample was 18.38. Gradient boosting achieved the lowest ASAM (ASAM=5.70) followed by logistic regression (ASAM=6.20), then random forest (ASAM=8.01). The estimated causal risk differences were -0.09 (95%PI: -0.17, -0.01) for gradient boosting, -0.09 (95%PI: -0.17, 0.00) for logistic regression and -0.15 (95%PI: -0.27, -0.02) for random forest.
Conclusion
Gradient boosting outperformed logistic regression in covariate balance. Our work supports the role of machine learning over traditional methods in obtaining more balanced datasets, resulting in more trustworthy estimates of the causal effect of public health interventions.
People recently released from prison experience poor health outcomes and evidence-based public health policy is critical to improve their health. Causal evidence for these policies can be found when exposure groups are balanced on all measured covariates, as in a randomised controlled trial. In observational studies, propensity scores are estimated to reweight data to achieve covariate balance. Using data from an observational study of incarcerated men, we compared how machine learning and traditional logistic regression propensity estimation methods perform in obtaining balanced datasets.
Methods
We evaluated three propensity estimation methods: logistic regression, gradient boosting and random forest. The resulting covariate balance was assessed using the average standardised absolute mean difference (ASAM; a lower ASAM indicates better balance between groups). For each method, we fit a causal model with inverse probability weighting to estimate causal risk difference and 95% prediction interval (95%PI). Data were from an observational cohort study of imprisoned men with survey and administrative data pre- and post-release. The exposure was self-reported access to emotional support whilst in prison (yes/no). The outcome was emergency department (ED) presentation within 100 days post prison release (yes/no), derived from administrative data.
Results
Of 326 men, 229 (70%) reported accessing emotional support whilst imprisoned; 75 (23%) attended ED within 100 days post release. ASAM in the unweighted sample was 18.38. Gradient boosting achieved the lowest ASAM (ASAM=5.70) followed by logistic regression (ASAM=6.20), then random forest (ASAM=8.01). The estimated causal risk differences were -0.09 (95%PI: -0.17, -0.01) for gradient boosting, -0.09 (95%PI: -0.17, 0.00) for logistic regression and -0.15 (95%PI: -0.27, -0.02) for random forest.
Conclusion
Gradient boosting outperformed logistic regression in covariate balance. Our work supports the role of machine learning over traditional methods in obtaining more balanced datasets, resulting in more trustworthy estimates of the causal effect of public health interventions.
Dr Jennifer Dunne
Research Fellow
Curtin University
External Validation of Dementia Risk Models in an Italian Community Cohort Study
Abstract
Background: Several dementia risk prediction models have demonstrated moderate to high predictive accuracy in their development cohorts. However, model performance in external validation studies shows high heterogeneity, often with poor transportability across different populations, healthcare systems and geographical settings. This study evaluated the predictive accuracy of dementia risk models when externally validated in an Italian setting.
Methods: Sixty dementia risk prediction models (identified from 39 studies) were externally validated using a longitudinal epidemiological cohort of community-dwelling older adults residing in Italy (n=1,453 participants; aged ≥65 years). All-cause dementia and Alzheimer disease (AD) were assessed over a 20-year period. Models were tested with Cox regression and discriminatory ability assessed using c-statistics with 95% confidence intervals (CIs).
Results: Among the 1,418 participants (Mean ± SD: 67.76 ± 15.65 years; female 51.39%) enrolled at baseline without dementia, 3.7% (n=53) developed dementia (including n=18 with AD), over a mean follow-up of 11.8 ± 5.62 years. Half (n=30) of the models could be fully validated. For partially validated models, the most common missing variable was genetic (i.e., Apolipoprotein E4) status. The top performing models were the Brief Dementia Screening Index (BDSI) and the eRADAR, with high performance (c-statistics: >0.80). The remaining fully validated models demonstrated low (c-statistic: 0.50-0.60) to moderate (c-statistic: 0.60-0.75) predictive accuracy.
Conclusion: Some of the included models showed robust external validity in our Italian cohort, particularly those that incorporated health and lifestyle risk factors (e.g., physical activity, smoking status, diet, and cardiovascular health). However, standardisation of risk factor measurement and classification is critically needed to enable comprehensive evaluation of prediction models across settings (e.g., consistent definitions of hypertension thresholds, uniform categorisation of dietary intake). Implementation of well-validated risk models could enable early intervention and risk modification, potentially reducing the substantial disease burden associated with dementia.
Methods: Sixty dementia risk prediction models (identified from 39 studies) were externally validated using a longitudinal epidemiological cohort of community-dwelling older adults residing in Italy (n=1,453 participants; aged ≥65 years). All-cause dementia and Alzheimer disease (AD) were assessed over a 20-year period. Models were tested with Cox regression and discriminatory ability assessed using c-statistics with 95% confidence intervals (CIs).
Results: Among the 1,418 participants (Mean ± SD: 67.76 ± 15.65 years; female 51.39%) enrolled at baseline without dementia, 3.7% (n=53) developed dementia (including n=18 with AD), over a mean follow-up of 11.8 ± 5.62 years. Half (n=30) of the models could be fully validated. For partially validated models, the most common missing variable was genetic (i.e., Apolipoprotein E4) status. The top performing models were the Brief Dementia Screening Index (BDSI) and the eRADAR, with high performance (c-statistics: >0.80). The remaining fully validated models demonstrated low (c-statistic: 0.50-0.60) to moderate (c-statistic: 0.60-0.75) predictive accuracy.
Conclusion: Some of the included models showed robust external validity in our Italian cohort, particularly those that incorporated health and lifestyle risk factors (e.g., physical activity, smoking status, diet, and cardiovascular health). However, standardisation of risk factor measurement and classification is critically needed to enable comprehensive evaluation of prediction models across settings (e.g., consistent definitions of hypertension thresholds, uniform categorisation of dietary intake). Implementation of well-validated risk models could enable early intervention and risk modification, potentially reducing the substantial disease burden associated with dementia.
Dr Jack Janetzki
Lecturer In Pharmacy And Pharmacology
University Of South Australia
Large-scale evidence: novel epidemiological approach to assess antibiotic risk of aortic events
Abstract
Title: Large-scale evidence: novel epidemiological approach to assess antibiotic risk of aortic events
Background:
Medicine regulators have warned of a potential link between fluoroquinolone (FQ) antibiotics and aortic aneurysm or dissection (AA/AD), but evidence remains inconclusive due to inconsistent study designs, exposure definitions, outcome identification, and confounding adjustments.
Aim: To assess AA/AD risk following FQ exposure using a novel distributed network analysis, compared to trimethoprim (TMP) and cephalosporins (CPH) for urinary tract infections (UTIs).
Methods:
We conducted a cohort study of patients aged ≥35 years initiating UTI antibiotics (2010–2019). Data from fourteen databases from five countries, standardised to the OMOP Common Data Model contributed to this study. The primary outcome was hospital admission for AA/AD within 90 days.
To account for confounding, a large-scale propensity score (PS) model was developed using regularised regression, incorporating baseline covariates. Target and comparator cohorts were matched based on the PS in a 1:1 ratio. A series of objective diagnostics were used to determine potential for bias after PS matching including, covariate balance, and clinical equipoise. Potential for systematic error was examined using 50 negative control experiments. Hazard ratios (HRs) from individual databases were pooled using Bayesian meta-analysis.
Results:
No increased AA/AD risk was observed for FQ versus TMP (pooled HR 0.91, 95% CI 0.73–1.10) or CPH (pooled HR 1.01, 95% CI 0.82–1.25). While patient characteristics and treatment patterns varied, treatment effect estimates showed minimal heterogeneity.
Conclusion:
This large-scale, multi-database analysis found no association between FQ use and AA/AD risk in patients with UTI. Our approach enables rapid, data-driven medicine safety assessments on a global scale.
Background:
Medicine regulators have warned of a potential link between fluoroquinolone (FQ) antibiotics and aortic aneurysm or dissection (AA/AD), but evidence remains inconclusive due to inconsistent study designs, exposure definitions, outcome identification, and confounding adjustments.
Aim: To assess AA/AD risk following FQ exposure using a novel distributed network analysis, compared to trimethoprim (TMP) and cephalosporins (CPH) for urinary tract infections (UTIs).
Methods:
We conducted a cohort study of patients aged ≥35 years initiating UTI antibiotics (2010–2019). Data from fourteen databases from five countries, standardised to the OMOP Common Data Model contributed to this study. The primary outcome was hospital admission for AA/AD within 90 days.
To account for confounding, a large-scale propensity score (PS) model was developed using regularised regression, incorporating baseline covariates. Target and comparator cohorts were matched based on the PS in a 1:1 ratio. A series of objective diagnostics were used to determine potential for bias after PS matching including, covariate balance, and clinical equipoise. Potential for systematic error was examined using 50 negative control experiments. Hazard ratios (HRs) from individual databases were pooled using Bayesian meta-analysis.
Results:
No increased AA/AD risk was observed for FQ versus TMP (pooled HR 0.91, 95% CI 0.73–1.10) or CPH (pooled HR 1.01, 95% CI 0.82–1.25). While patient characteristics and treatment patterns varied, treatment effect estimates showed minimal heterogeneity.
Conclusion:
This large-scale, multi-database analysis found no association between FQ use and AA/AD risk in patients with UTI. Our approach enables rapid, data-driven medicine safety assessments on a global scale.
Dr Serah Kalpakavadi
PhD Student
University Of Tasmania
Case crossover design in data linkage studies: A practical approach in epidemiology
Abstract
Introduction
Case-crossover designs are useful in epidemiology for investigating the effects of transient or proximal exposures on acute events. Here we describe our methods for this design using data linkage to examine the association between hospital encounters and risk of first ever stroke in Tasmania, Australia.
Methods
We used a linked dataset encompassing all public hospital admissions, Emergency Department [ED] presentations and deaths from 2007-2020. First-ever strokes and hospital presentations were identified from discharge diagnoses using ICD-10 AM codes. A case-crossover study design, in which each case served as his/her own control, examined the association between hospital encounters as the exposure and first-ever strokes as the outcome within 90 days. Conditional logistic regression modelled the associations. Time-invariant covariates are self-controlled by the design, as the case periods (across 1-90 days) before stroke and the control periods 1 year (across 366-455 days) before stroke are within the same individual.
Results
There were 207,840 records in our linked dataset with 15,117 stroke events. 4,907 first-ever stroke events were identified during 2015-2020, using an eight-year lookback period. Twenty-five percent (n=1250) had hospital encounters in the 90 days before stroke. We found high odds of stroke associated with hospital encounters in the 90 days before stroke (odds ratio: 2.64 95% CI: 2.34-2.98), compared to hospital encounters in the control period 1 year before stroke. Benefits of this design include self-adjustment for time-invariant confounders and limiting recall bias due to use of validated ICD-10 AM codes for identifying exposures and outcomes in the linked dataset. Limitations include lack of control of time varying confounders, specificity of ICD coding and complexity of patient journeys to classify hospital encounters in a large dataset.
Conclusion
We demonstrate how a case-crossover study can be conducted using a data linkage study, where a control population may be difficult to source.
Case-crossover designs are useful in epidemiology for investigating the effects of transient or proximal exposures on acute events. Here we describe our methods for this design using data linkage to examine the association between hospital encounters and risk of first ever stroke in Tasmania, Australia.
Methods
We used a linked dataset encompassing all public hospital admissions, Emergency Department [ED] presentations and deaths from 2007-2020. First-ever strokes and hospital presentations were identified from discharge diagnoses using ICD-10 AM codes. A case-crossover study design, in which each case served as his/her own control, examined the association between hospital encounters as the exposure and first-ever strokes as the outcome within 90 days. Conditional logistic regression modelled the associations. Time-invariant covariates are self-controlled by the design, as the case periods (across 1-90 days) before stroke and the control periods 1 year (across 366-455 days) before stroke are within the same individual.
Results
There were 207,840 records in our linked dataset with 15,117 stroke events. 4,907 first-ever stroke events were identified during 2015-2020, using an eight-year lookback period. Twenty-five percent (n=1250) had hospital encounters in the 90 days before stroke. We found high odds of stroke associated with hospital encounters in the 90 days before stroke (odds ratio: 2.64 95% CI: 2.34-2.98), compared to hospital encounters in the control period 1 year before stroke. Benefits of this design include self-adjustment for time-invariant confounders and limiting recall bias due to use of validated ICD-10 AM codes for identifying exposures and outcomes in the linked dataset. Limitations include lack of control of time varying confounders, specificity of ICD coding and complexity of patient journeys to classify hospital encounters in a large dataset.
Conclusion
We demonstrate how a case-crossover study can be conducted using a data linkage study, where a control population may be difficult to source.
Dr Khai Lin Kong
MPH student/Infectious Diseases Physician
Melbourne School of Population and Global Health, University of Melbourne/Monash Health
Dynamic Time Warping and the spatiotemporal variation of COVID-19 case time series
Abstract
Background: Analysing the spatiotemporal variation of COVID-19 disease activity can identify underlying COVID-19 transmission dynamics and inform targeted public health responses and interventions. In this study, we explored the use of Dynamic Time Warping (DTW), a time series analysis method, to investigate the spatiotemporal variation in COVID-19 disease activity in the United Kingdom (UK) from 01-11-2020 to 19-5-2022.
Methods: We performed DTW analysis on 380 COVID-19 case time series from 380 Lower Tier Local Authorities (LTLA) in the UK. Using DTW distance as input, we performed hierarchical clustering analysis (HCA) of these time series to investigate their similarity. We inferred the lead-lag time relationship amongst these time series using relative time lags (RTLs), focusing on three periods reflecting the emergence of Alpha, Delta and Omicron BA.1 variants of SARS-CoV-2. We analysed the lead-lag time relationship separately for each nation (England, Scotland, Wales and Northern Ireland).
Results: All case time series within each of Scotland and Wales were found to be closely related. We observed strong spatial clustering of case time series from LTLAs in England. The lead-lag time analysis in England showed that LTLAs in southeast England led the Alpha wave, LTLAs in Manchester led the Delta wave, and LTLAs in London led the Omicron BA.1 wave.
Conclusion: We identified spatiotemporal clustering of COVID-19 case time series in the UK using DTW analysis. The results of our lead-lag time analysis correspond to the findings from previous phylogeographic studies. DTW has the potential to describe spatiotemporal variation of infectious diseases such as COVID-19. High-quality epidemiological data and further studies to determine DTW’s optimal settings are critical to maximising its potential in epidemic time series analysis.
Methods: We performed DTW analysis on 380 COVID-19 case time series from 380 Lower Tier Local Authorities (LTLA) in the UK. Using DTW distance as input, we performed hierarchical clustering analysis (HCA) of these time series to investigate their similarity. We inferred the lead-lag time relationship amongst these time series using relative time lags (RTLs), focusing on three periods reflecting the emergence of Alpha, Delta and Omicron BA.1 variants of SARS-CoV-2. We analysed the lead-lag time relationship separately for each nation (England, Scotland, Wales and Northern Ireland).
Results: All case time series within each of Scotland and Wales were found to be closely related. We observed strong spatial clustering of case time series from LTLAs in England. The lead-lag time analysis in England showed that LTLAs in southeast England led the Alpha wave, LTLAs in Manchester led the Delta wave, and LTLAs in London led the Omicron BA.1 wave.
Conclusion: We identified spatiotemporal clustering of COVID-19 case time series in the UK using DTW analysis. The results of our lead-lag time analysis correspond to the findings from previous phylogeographic studies. DTW has the potential to describe spatiotemporal variation of infectious diseases such as COVID-19. High-quality epidemiological data and further studies to determine DTW’s optimal settings are critical to maximising its potential in epidemic time series analysis.
Mrs Negin Maroufi
Student
University Of Otago (Wellington)
Choosing Surveillance Systems that Best Support Machine Learning for Short-term Influenza Forecasting
Abstract
Background
Influenza surveillance is critical for supporting a timely public health response to influenza outbreaks and seasonal peaks. However, existing systems are often not optimised for predictive modelling. Given the growing role of Artificial Intelligence (AI) and Machine Learning (ML) in modelling future short-term disease scenarios, surveillance systems need to be selected and adapted to effectively support these applications. This study proposes a framework, based on key characteristics required for short-term forecasts, to evaluate and then enhance surveillance systems for AI/ML-driven forecasting, using New Zealand as a case study.
Methods
This framework focuses on eight key attributes—timeliness, sensitivity, specificity, representativeness, coverage, robustness, completeness, and historical data—selected from 16 data quality-related attributes, in a broader pool of 31. Attributes were chosen based on expert input, AI/ML requirements, and established evaluation frameworks. A comprehensive review of government reports, official data, and literature was undertaken to characterise New Zealand’s influenza surveillance systems, with weighted scoring used to evaluate their suitability for model training and short-term forecasting.
Results
In New Zealand, one sentinel community-based surveillance system (SHIVERS) and one hospital-based system (SARI) emerged as the most suitable surveillance systems for AI/ML-based predictive models in both training and forecasting. While the national hospital discharge system (NMDS) and national mortality database offer strong potential for training, their delayed reporting limits short-term forecasting. Laboratory-based virological surveillance plays a pivotal role in bridging community and hospital data, enhancing model accuracy by integrating virological confirmation with broader surveillance insights.
Conclusions
This study highlights gaps where surveillance systems could be improved for better predictive modelling. Enhancing real-time data collection and leveraging multiple data sources could improve forecasting accuracy, helping to make timely, more informed decisions for managing influenza outbreaks and seasonal peaks in healthcare demand.
Keywords: Influenza, Surveillance, Artificial Intelligence, Machine Learning, Short-term Forecasting.
Influenza surveillance is critical for supporting a timely public health response to influenza outbreaks and seasonal peaks. However, existing systems are often not optimised for predictive modelling. Given the growing role of Artificial Intelligence (AI) and Machine Learning (ML) in modelling future short-term disease scenarios, surveillance systems need to be selected and adapted to effectively support these applications. This study proposes a framework, based on key characteristics required for short-term forecasts, to evaluate and then enhance surveillance systems for AI/ML-driven forecasting, using New Zealand as a case study.
Methods
This framework focuses on eight key attributes—timeliness, sensitivity, specificity, representativeness, coverage, robustness, completeness, and historical data—selected from 16 data quality-related attributes, in a broader pool of 31. Attributes were chosen based on expert input, AI/ML requirements, and established evaluation frameworks. A comprehensive review of government reports, official data, and literature was undertaken to characterise New Zealand’s influenza surveillance systems, with weighted scoring used to evaluate their suitability for model training and short-term forecasting.
Results
In New Zealand, one sentinel community-based surveillance system (SHIVERS) and one hospital-based system (SARI) emerged as the most suitable surveillance systems for AI/ML-based predictive models in both training and forecasting. While the national hospital discharge system (NMDS) and national mortality database offer strong potential for training, their delayed reporting limits short-term forecasting. Laboratory-based virological surveillance plays a pivotal role in bridging community and hospital data, enhancing model accuracy by integrating virological confirmation with broader surveillance insights.
Conclusions
This study highlights gaps where surveillance systems could be improved for better predictive modelling. Enhancing real-time data collection and leveraging multiple data sources could improve forecasting accuracy, helping to make timely, more informed decisions for managing influenza outbreaks and seasonal peaks in healthcare demand.
Keywords: Influenza, Surveillance, Artificial Intelligence, Machine Learning, Short-term Forecasting.
Ms Tu Nguyen
PhD Candidate
Murdoch Children's Research Institute
SnotWatch: spatiotemporal ecological platform for understanding viral contribution to important health outcomes
Abstract
BACKGROUND: Increasingly, infectious risk factors have been identified as key drivers of the burden of important health conditions such as cardiovascular disease, cancers and chronic kidney diseases. Estimating the burden attributable to specific infectious pathogens is vital to inform public health decision-making, but hampered by lack of representative population data. SnotWatch was established in 2019 as an ecological data platform for real-time population-level analyses of spatiotemporal associations between viral activity and important disease outcomes in Victoria.
METHODS: SnotWatch uses de-identified viral data with contemporaneous health outcome data to examine associations. The platform has an ongoing data feed of results from laboratory polymerase chain reaction (PCR) tests from eight major pathology services in Victoria performed from 2010 onwards. Spatiotemporal analyses, incorporating generalized linear modelling and fit testing are used to determine the relationship between viral activity and disease outcomes, such as state-wide hospital admissions or emergency department presentations. Population attributable burden may be quantified with appropriate exposure-response functions.
RESULTS: SnotWatch established a databank of over 3 million respiratory PCR tests from laboratories servicing Victoria, providing comprehensive coverage of respiratory viral activity in Victoria. The model has successfully detected associations between febrile seizures and paediatric hepatitis with respiratory viruses, chilblains with COVID-19 and febrile seizures with human metapneumovirus. Incorporating area-level environmental exposure data, such as air pollution and climate, is being incorporated into SnotWatch to adjust for multiple population-level factors to improve attributable burden estimates. As the platform matures, continued collaboration with laboratory services will facilitate improved data on viral activity and, in turn, the modelling of important health conditions.
CONCLUSION: Understanding the burden attributable to specific infectious pathogens are integral to inform public health practice. SnotWatch provides a foundation for exploring associations between viral activity and health conditions that could not be readily quantified at a population level.
METHODS: SnotWatch uses de-identified viral data with contemporaneous health outcome data to examine associations. The platform has an ongoing data feed of results from laboratory polymerase chain reaction (PCR) tests from eight major pathology services in Victoria performed from 2010 onwards. Spatiotemporal analyses, incorporating generalized linear modelling and fit testing are used to determine the relationship between viral activity and disease outcomes, such as state-wide hospital admissions or emergency department presentations. Population attributable burden may be quantified with appropriate exposure-response functions.
RESULTS: SnotWatch established a databank of over 3 million respiratory PCR tests from laboratories servicing Victoria, providing comprehensive coverage of respiratory viral activity in Victoria. The model has successfully detected associations between febrile seizures and paediatric hepatitis with respiratory viruses, chilblains with COVID-19 and febrile seizures with human metapneumovirus. Incorporating area-level environmental exposure data, such as air pollution and climate, is being incorporated into SnotWatch to adjust for multiple population-level factors to improve attributable burden estimates. As the platform matures, continued collaboration with laboratory services will facilitate improved data on viral activity and, in turn, the modelling of important health conditions.
CONCLUSION: Understanding the burden attributable to specific infectious pathogens are integral to inform public health practice. SnotWatch provides a foundation for exploring associations between viral activity and health conditions that could not be readily quantified at a population level.
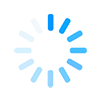